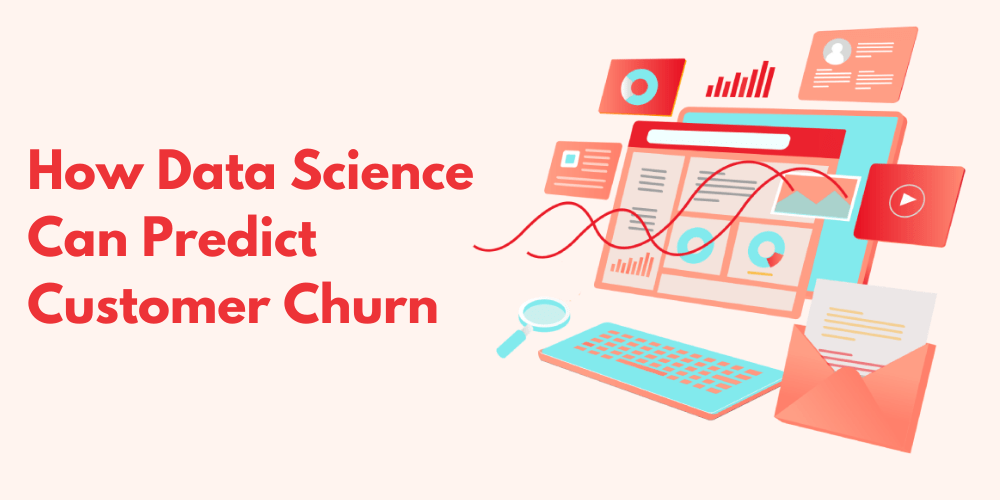
Curious About Churn Models? Here Are Some Answers.
How do I find the right data scientist or analytics firm to help me?
What Can Data Science Do for Your Company?
The churn rate refers to the number of customers who discontinue using your product or service within a specified period of time. This period of time can be as short as two weeks or as long as six months. Your business’s model and strategy play a role here, as well.
Your customer churn directly affects the amount of revenue you lose in a given time period. Having a paid service that churns monthly is very different from having a free service that churns monthly.
While a 5% decline in free customers does not necessarily imply a drop in revenue, a 10% drop in paid customers could result in a 20% drop in revenue generated.
A data science consultant can better identify your problem and help you improve your customer retention efforts if you know how much revenue you’re losing because of customer churn.
Data scientists use churn models to identify the likelihood that a customer will leave.
The customer churn model takes into account factors such as customer purchase intervals, cancellations, follow-up calls, and emails, and on-page engagement to predict when a customer will leave. This is usually done by assigning each customer a personal score.
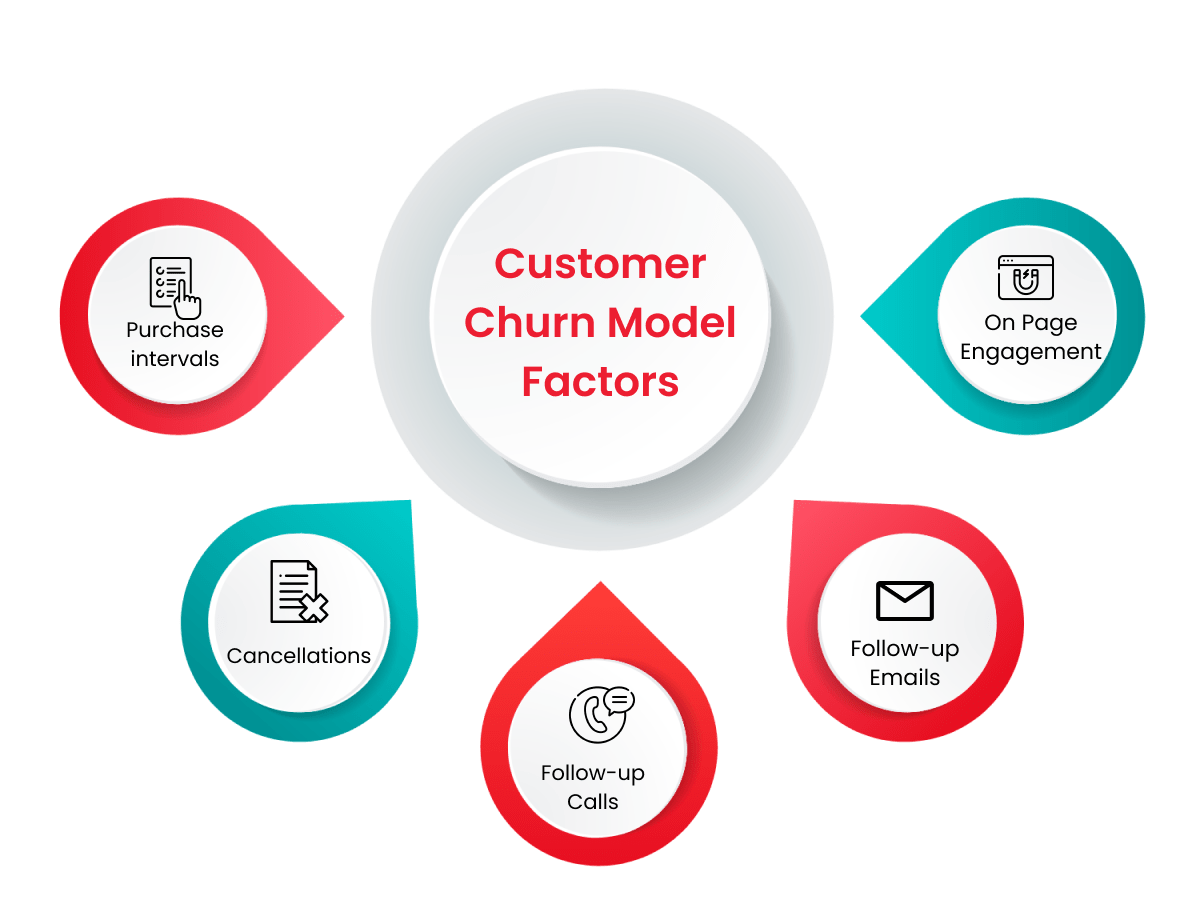
What's the Best Case Scenario for a Churn Model?
A customer retention strategy is the ideal outcome of a churn prediction model. Every department that has been implicated in the customer loss must work together to find a solution if a retention strategy is to be successful.
It is common for customer churn models to fail if cross-department cooperation is lacking. This could be due to the fact that the data scientist didn’t take the time to understand the churn situation before creating the model. A lack of access to relevant data, overly-complicated math (especially if the word “neural net” is used), or an employee’s fear of losing their job may have prevented a data scientist from being fully informed of the problem.
Your data scientist should interact with everyone in your company to ensure that the model succeeds. If you want your business to grow, you need data scientists who have a thorough understanding of the problem at hand.
Is a Churn Model Guaranteed to Be a Success?
Definitely. If you want to improve customer retention and minimize customer churn, consider the following suggestions:
- Make sure that your company is collecting and labeling all of the relevant data.
- Prior to developing a solution, hold several meetings with a data scientist to discuss the problem.
- Before building the full system, have the data scientist create a clear proof of concept model.
- Explain to your team how the model will be used and what they can expect.